A large language model (LLM), like a chatbot, is not responsible for its own actions. Nor is it a separate legal entity. Air Canada discovered this the hard way when its chatbot misinformed passenger Jake Moffatt about the airline’s refund policies. When he applied for his partial refund, Air Canada declined. It argued that the correct information was available elsewhere on its website and, furthermore, the chatbot was “a separate legal entity” the company was not responsible for.
Things took a turn for the litigious. The judge dismissed the idea that the chatbot was simply an unruly independent contractor.
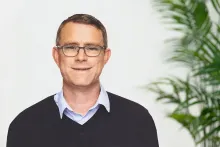
The whole debacle got us thinking more deeply about the data we generate at an ever-faster pace, especially as we now bare our souls to local and cloud-based large LLMs and associated systems.
In the same way that businesses initially adopted cloud technologies (both formally and informally), the use of LLMs is set to have a wide-reaching impact on the execution of critical processes related to legal data requests.
Ease of access
The digital workplace has only just started to transform thanks to the integration of AI technologies into business operations, from cloud-based LLMs like Microsoft Copilot, OpenAI's ChatGPT, and Google's Gemini (previously Bard), to locally-run enterprise LLMs.
This transformation brings with it significant legal disclosure challenges and implications, especially as the volume of data generated by these AI tools becomes increasingly relevant to litigation.
The discoverability of AI-generated data
The use of AI technologies has made vast amounts of data potentially discoverable in litigation – including everything from casual conversations with AI chatbots to structured interactions with enterprise-grade LLMs.
Often encompassing sensitive or proprietary information, AI-generated data could be of upmost importance to organisations. This underscores the necessity of organisations taking control of their data retention policies and the mechanisms for accessing data when needed.
Understanding how to access, manage, and preserve data is paramount for legal compliance and risk management. To adapt a phrase, “the stable door is open, but the horse may not yet have bolted.” Organisations must act rapidly to secure the metaphorical horse. The first challenge is to understand the different types of LLMs that organisations may be using.
Cloud-based LLMs: Copilot, ChatGPT, and Gemini
Platforms like Microsoft Copilot, ChatGPT, and Google's Gemini exemplify the advancements in AI-powered communication and productivity tools. Each platform has its own approach to data retention and privacy:
- Microsoft Copilot operates within the Microsoft 365 ecosystem, adhering to established data management and retention policies (similar to those for Microsoft Teams).
- OpenAI's ChatGPT retains interactions for a limited period, emphasising user privacy. It also has an option to disable history, affecting how data is stored and used.
- Google's Gemini focuses on retaining contextual information, rather than full conversation transcript. It comes with specific provisions for Google Workspace users through Google Vault.
These varied approaches highlight the importance of understanding platform-specific data management practices, especially in preparing for potential eDiscovery requests.
Locally-run enterprise LLMs
For organisations deploying their own LLMs, the eDiscovery implications are equally significant. Data generated and stored locally falls under the company's direct control, necessitating robust data governance and retention policies.
Unlike cloud-based LLMs, where the service provider may offer tools and protocols for data management, enterprises must independently ensure their LLMs comply with legal and regulatory requirements.
Shadow IT and the playful professional
For many individuals, the buzz of generative AI creates a sense of insatiable curiosity. Professionals, from lawyers to accountants, are actively interested in – if not cajoled into – being ahead of the AI curve. Such usage may already breach existing acceptable use policies, but the temptation to stay ahead and experiment may lead to a clouding of judgment.
These professionals will typically be going beyond what your usual developer or incident response specialist would attempt to do with LLMs, playing with their own experiments in:
- using downloaded models;
- submitting confidential information to uncontrolled environments; and
- running local LLMs on company or personal hardware.
Typical software in use might be PrivateGPT, LM Studio, Fast Chat, GPT4All, OLlama as well as the aforementioned online tools (Gemini, ChatGPT and others such as Claude.ai)
All this presents employers with a host of latent risks – not least because some LLMs may breach the standards of acceptable data use, but also the movement of information outside of the corporate environment and into personal or cloud-based environments.
Strong policies and training updates are needed to encourage and enforce the centralisation of such experimentation, and to provide controlled test environments with carefully defined data retention requirements. This will mitigate the possibility of needing additional targeted collection during legal discovery of personal or business devices and ensure that staff know the risks inherent with working outside of the corporate environment.
The urgency of litigation holds
In the course of legal proceedings, the ability to quickly implement a litigation hold becomes critical. This legal mechanism requires organisations to preserve all relevant data, including AI-generated content, that might be subject to discovery.
The speed at which these holds must be enforced highlights the need for both proactive eDiscovery planning and understanding the technical capabilities of both cloud-based and local AI systems.
- Failure to act: Organisations risk sanctions and accusations of negligence if they don’t know how to enforce litigation holds on AI systems. Penalties range from fines to adverse judgments, which underscores the legal obligations companies face in managing digital evidence.
- Third-party and local retention policies: Understanding the retention policies of third-party AI providers, as well as managing local data storage practices, is essential for compliance. Differences in these policies can significantly impact an organisation's ability to respond to eDiscovery requests efficiently and effectively.
Minimising risks and ensuring compliance
The risk of sanctions for failing to comply with eDiscovery requirements emphasises the need for organisations to:
- Understand AI data management: how data is generated, stored, and can be accessed across all AI platforms in use.
- Implement robust data governance: develop and enforce data governance policies that include provisions for AI-generated data, ensuring readiness for litigation holds and eDiscovery requests.
- Train and prepare: equip legal and IT teams with the knowledge and tools necessary to respond promptly to litigation involving AI-generated data.
All of this is vital if digital forensics practitioners are to find what they need.
Future reproducibility
A pivotal, yet often overlooked, aspect of managing AI technologies within enterprises is the necessity of preserving the versioning of models and the software environments in which these LLMs operate.
This is a crucial consideration – not only for maintaining the integrity of AI-generated data, but also for ensuring that any responses or decisions made by AI can be accurately reproduced in the future. And this is a particularly pertinent scenario in litigation.
As AI models are continually updated and improved, the responses they generate can vary significantly from one version to another. This evolution, while beneficial for enhancing the model's accuracy and functionality, poses a challenge for eDiscovery, especially when specific interactions need to be reviewed or replicated years after they occurred.
Enterprises must therefore adopt strategies to preserve the versioning of AI models and the software environments that run them. This preservation is essential for several reasons:
- Digital forensics and litigation preparedness: The ability to reproduce past AI interactions as they originally occurred can be critical evidence in civil and criminal cases. Without accurate versioning and environmental data, it may be challenging to demonstrate the context and integrity of AI-generated responses, potentially undermining their admissibility or relevance in legal proceedings.
- Regulatory compliance: Beyond litigation, regulatory frameworks like GDPR, UK GDPR, and the forthcoming EU AI Act may require organisations to explain or justify decisions made with the assistance of AI. Preserving the specific versions of AI models used for decision-making is key to meeting these regulatory demands.
- Ethical and operational integrity: Maintaining a historical record of AI model versions and their operational environments reflects a commitment to transparency and the ethical use of AI. It enables organisations to review and learn from past interactions, ensuring AI technologies are deployed responsibly and effectively over time.
Strategies for preservation
To address these challenges, organisations should consider implementing comprehensive data governance policies that include:
- Version control systems: Use version control for AI models and their associated software environments to ensure that each state can be uniquely identified, stored, and retrieved.
- Archival solutions: Invest in robust archival solutions that can securely store not only the AI models and software versions, but also the data and interactions they have processed.
- Documentation and metadata: Maintain detailed documentation and metadata for AI interactions, including timestamps, model versions, and environmental conditions, to facilitate future retrieval and reproduction.
Evolving role of IT personnel
Alongside their legal counterparts, IT professionals play a pivotal role in navigating the intricacies of AI-generated data and eDiscovery preparedness. The following IT capabilities will be increasingly critical:
- Training: Specialised training on data preservation across a variety of AI platforms (Copilot, ChatGPT, local LLMs, etc.), with an emphasis on preserving the model's state and environmental conditions.
- Tooling: Familiarisation with both in-house and commercial tools dedicated to extracting, indexing, and securing AI-generated data relevant for legal holds. This may include eDiscovery tools with AI-specific extensions and purpose-built platforms for LLM version and output management.
- Collaboration: Establishing a regular forum for knowledge sharing and coordination between legal and IT teams to address the ongoing technological shifts within this arena.
Proactive AI data governance
The ever-expanding landscape of AI in the workplace can leave organisations floundering when it comes to managing and controlling AI-generated data.
Periodic eDiscovery readiness assessments, specifically focusing on AI-generated data, offer organisations multiple advantages. These assessments help to:
- identify gaps in policies;
- improve data management practices;
- reduce risk exposure; and
- ultimately increase the legal department's confidence should future litigation touch on the use of AI systems.
However, a proactive approach based on establishing rigorous AI data governance is fundamental. To mitigate the risks inherent in the AI era, a focus on the following foundational principles that set the course for resilient eDiscovery preparedness:
- Classification: Classify your AI data with clarity. Understand the distinction between transient interactions on conversational AI platforms and AI-generated contracts or financial reports. Privacy requirements, commercial sensitivity and the business value of the data should guide how it’s stored, secured, and retained.
- Retention: Establish well-defined, legally defensible retention policies specific to AI data. Balancing potential regulatory requirements with organisational needs is vital. This ensures you're neither holding onto unnecessary data (data hoarding exposes you to liability) nor prematurely deleting information that may be discoverable later.
- Access control: Determine who within your organisation has access to AI data and is responsible for safeguarding highly sensitive information. Secure your AI systems and their outputs with encryption and robust access controls, which will minimise risk and the potential for data misuse.
- Monitoring: Implementing regular tracking and monitoring mechanisms for AI systems can be crucial in the aftermath of incidents. These might include audit logs and usage data that allow for forensic analysis and can clarify interactions in the event of litigation.
- Frameworks: While building these policies may seem daunting, established frameworks provide crucial guidance for enterprises seeking to ensure AI data is aligned with both ethical and regulatory concerns. Referencing the NIST AI Risk Management Framework and the guidelines set out in the EU AI Act will provide a structured path toward robust AI governance policies.
Integrating eDiscovery with broader regulatory requirements
The complexities of eDiscovery in the era of AI are not standalone challenges, but are intricately linked with broader legislative frameworks that govern digital operations and data protection. Being on top of eDiscovery policies is essential to litigation preparedness and to ensuring compliance with critical regulations such as:
- the Digital Operational Resilience Act (DORA);
- the General Data Protection Regulation (GDPR) and its counterpart in the UK (UK GDPR); and
- the forthcoming EU AI Act.
These regulations mandate rigorous data management and protection practices, and emphasise the importance of privacy, transparency, and security in handling personal and sensitive information.
For instance, robust mechanisms for managing AI-generated content are mandated by GDPR and UK GDPR principles around data minimisation and the rights of individuals to access and request the deletion of their data. Similarly, the EU AI Act, with its focus on ensuring the safe and ethical use of AI, underscores the need for transparency in AI operations, including how AI-generated data is stored, used, and made discoverable.
Conclusion: the strategic imperative of compliance and eDiscovery readiness
As AI technologies continue to reshape business communications and data generation, the dynamic landscape of eDiscovery demands a proactive and informed approach. Organisations must navigate the discoverability of AI-generated data with a keen understanding of their legal obligations and the technical capabilities of AI systems. In addition, ensuring the future reproducibility of AI-generated answers through careful preservation of model versioning and software environments, like saving the ‘blueprints of a building’ rather than just the ‘construction materials’.
By proactively managing data retention policies, preparing for litigation holds, and understanding the potential risks of non-compliance, companies mitigate the challenges posed by eDiscovery and, as a bonus, also align with the broader objectives of regulations like DORA, GDPR, UK GDPR, and the EU AI Act.
This holistic approach to data governance and AI management is essential for maintaining operational integrity, ensuring data protection, and upholding the ethical standards expected in the digital age.
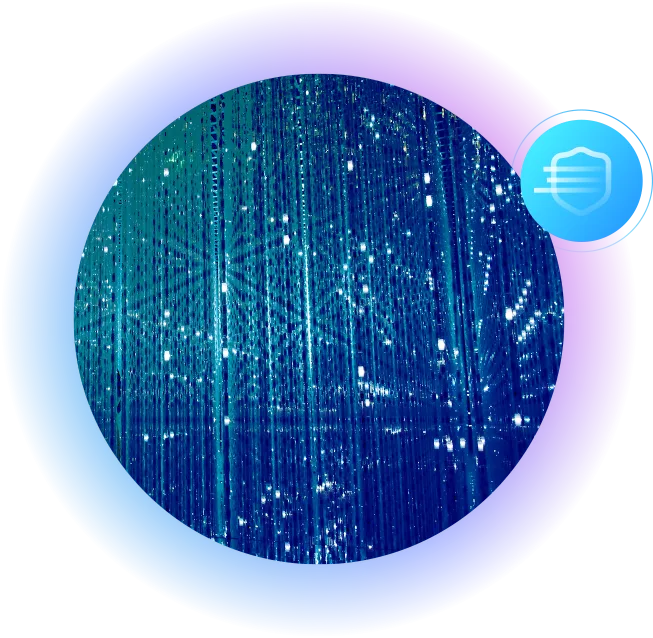
Cyber Risk
We bring the best of our collective experience, energy and creative power to fiercely safeguard our clients and fortify their communities.
We safeguard clients and their communities
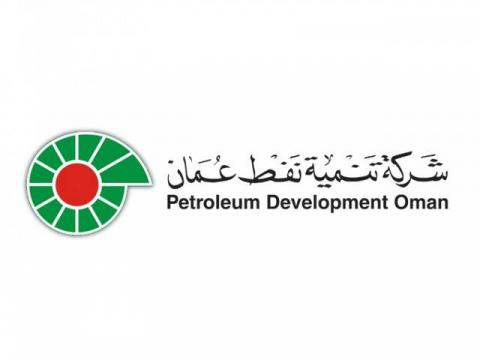
Petroleum Development Oman Pension Fund
“Thomas Murray has been a very valuable partner in the selection process of our new custodian for Petroleum Development Oman Pension Fund.”
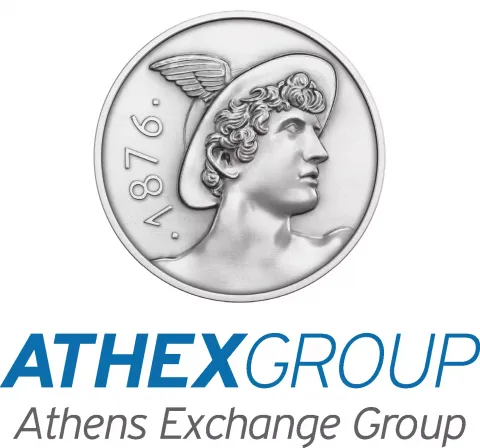
ATHEX
"Thomas Murray now plays a key role in helping us to detect and remediate issues in our security posture, and to quantify ATHEX's security performance to our directors and customers."
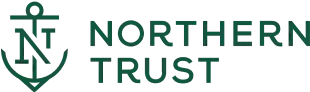
Northern Trust
“Thomas Murray provides Northern Trust with a range of RFP products, services and technology, delivering an efficient and cost-effective solution that frees our network managers up to focus on higher Value activities.”
Insights
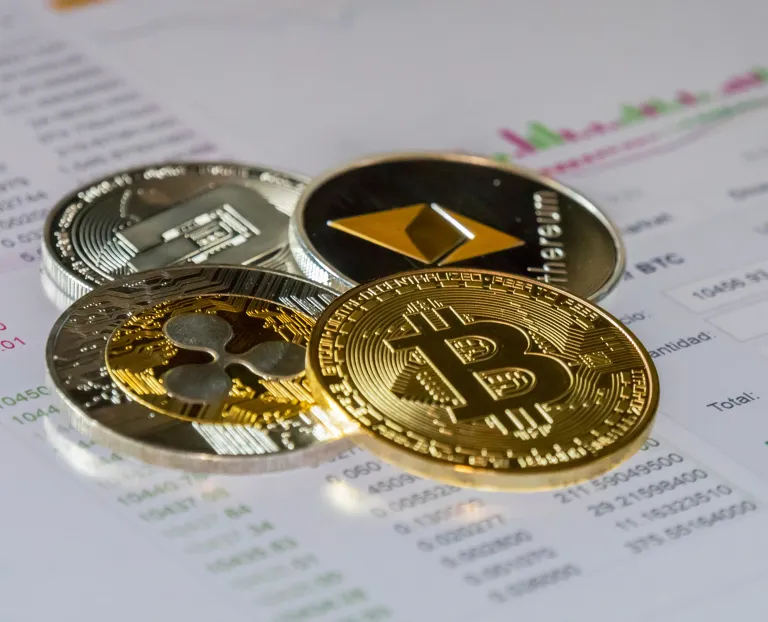
What Is Digital Asset Custodian Monitoring (DACM)?
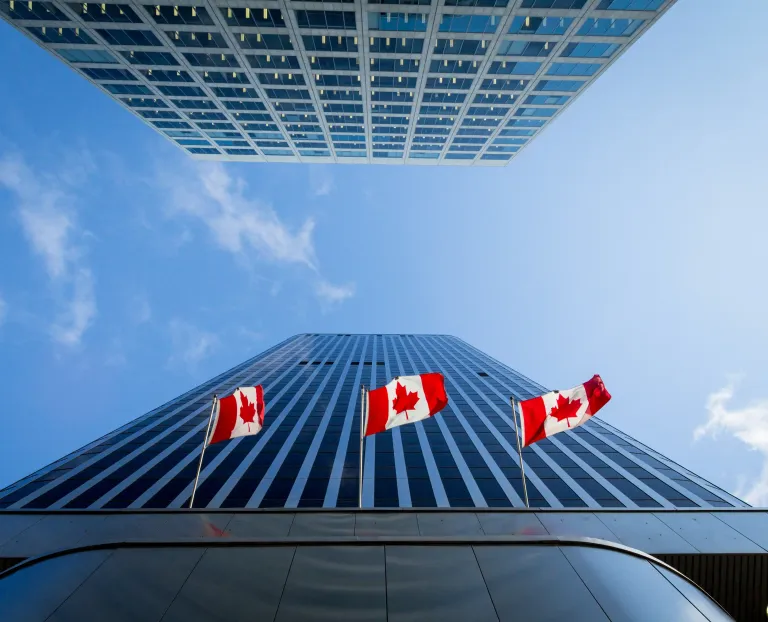
Operational Due Diligence and Regulations in Canada
As regulators continue to strengthen their focus on operational risk management, Operational Due Diligence (ODD) has become crucial for Canadian funds
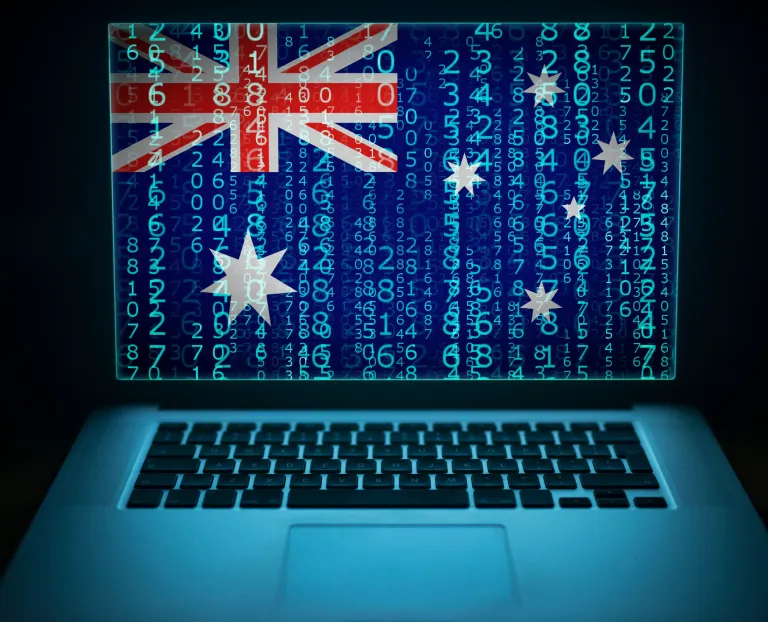
Recent Cyber Attacks on Australian Super Funds: A Wake-Up Call for Enhanced Cyber Security
Recent cyber attacks on Australia’s Super funds highlight the need for stronger security.
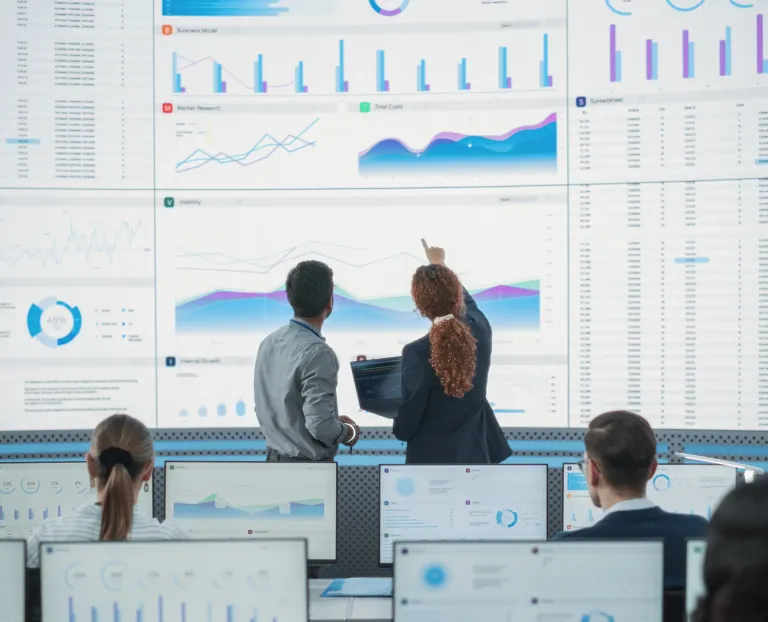
The Rising Threat: How Cyber Risk is Reshaping Operational Due Diligence Priorities
Cyber threats are reshaping operational due diligence. Learn how investors are adapting to evolving risks and protecting their portfolios.